Mathematical models of cancer evolution and cancer therapy
A significant part of our research program has been dedicated to investigate cellular evolutionary dynamics to understand how cancer initiates and progresses, and how treatment approaches can be improved such that tumor evolution towards a resistant state is prevented. This work spans a broad range of topics, including the dynamics of treatment responses, the evolution of drug resistance, the role of genetic instability for cancer initiation, the dynamics of stem cells, the evolution of escape from feedback control, and the role of feedback regulatory processes in carcinogenesis cancer therapy. Some of this work is described in the books “Dynamics of Cancer: Mathematical Foundations of Oncology” and “Targeted Cancer Treatment in Silico”. As an example, the following summarizes some of our work related to the treatment of leukemias.
Recently, our research focused on the treatment of chronic lymphocytic leukemia (CLL) with the tyrosine kinase inhibitor ibrutinib. In collaboration with Dr Jan Burger (MD Anderson Cancer Center), we developed mathematical approaches to quantitatively characterize the treatment response of CLL to ibrutinib, and to obtain patient-specific estimates for crucial kinetic parameters. This work has resulted in a number of novel insights: (i) By using mathematical models to quantify the death rate of CLL cells in blood and the tissue compartments during ibrutinib therapy, we found that a substantial amount of cell death occurs in the tissue compartments. It was previously believed that cell death mostly occurred in the blood compartment, once cells redistributed from the tissue to the blood during treatment. (ii) Parameter estimation allowed us to calculate the percentage of the tissue tumor burden that redistributed from the tissue to the blood during treatment, which was previously thought to be substantial. We found, however, that only a small fraction of the total tissue tumor cells redistributed to the blood during therapy, and that the majority of the cells died in the tissue. (iii) We found an interesting correlation between traditional risk factors and treatment responses among the patient cohort. Significantly faster treatment-induced cell death rates were measured in patients that were characterized by “high-risk” IGHV unmutated CLL (which was typically associated with poor prognosis in the context of chemotherapy), than in “lower risk” IGHV mutated CLL. Hence, the prognostic meaning of traditional risk factors might have to be re-evaluated in the context of newer treatments. (iv) Based on these parameter estimates, we subsequently built a mathematical framework to study the evolution of drug resistance in CLL treated with ibrutinib. Such models can be used to calculate personalized predictions about disease relapse times under different treatment schedules, based on patient-specific parameter estimates. We are currently developing this further by analyzing the dynamics of disease relapse in a patient cohort to test the predictive power of this approach.





Dynamics of viruses and immune responses
We have a long-standing interest in understanding the dynamics of virus infections in vivo, and their interactions with cytotoxic T lymphocyte (CTL) responses, an important branch of the adaptive immune system to fight viral infections. We have performed a large body of work in this area of research, including topics concerned with CTL memory, virus dynamics in murine infections, virus replication in CD4 T helper deficient hosts, and interactions between HIV and immune responses. A common theme is the application of mathematical models to experimental data to interpret observations and to test mathematical predictions. Some of this work is summarized in my book “Killer Cell Dynamics”.
A recent interest of the lab has been the direct cell-to-cell transmission of HIV-1 and the consequences for virus dynamics. HIV-1 can spread through its target cell population in two ways (1) During free virus transmission, offspring virus particles are released into the extracellular environment, and subsequently can infect new target cells upon contact. (2) In contrast, during direct cell-to-cell transmission, an infected cell connects with a target cell through the formation of virological synapses, which results in the simultaneous transfer of multiple viruses to the target cell (synaptic transmission). The most basic question concerns the relative contribution of free virus and synaptic transmission to virus growth in its target cell population. In a series of papers, we developed detailed mathematical models that take into account both modes of virus transmission. In collaboration with Dr David Levy (NYU), the models were applied to in vitro experimental data to estimate parameters, which showed that free virus and synaptic transmission contribute approximately equally to virus spread. In collaboration with Dr Benjamin Chen’s lab at Mount Sinai, we used mathematical models to investigate how common the co-transmission of different virus strains occurs through virological synapses in vivo. We applied our models to an experimental system in which humanized mice were infected with reporter viruses labeled with different fluorescent colors. This work suggests a relatively frequent occurrence of viral co-transmission, indicating that synaptic transmission plays an important role for evolutionary dynamics in vivo, particularly in the context of recombination. This collaboration further demonstrated micro-anatomical clustering of viral types within lymphoid tissues, through the application of mathematical techniques to experimental data. This implies that viral spread is driven by local processes and not by a diffuse viral cloud.
Treatment of cancers with oncolytic viruses
Oncolytic viruses have been engineered to specifically infect cancer cells, but not healthy cells. They replicate in the cancer cells, and spread the infection to further cancer cells. The idea is to infect a patient with such viruses with the aim to drive the tumor into remission and to also attack metastatic lesions. Many promising results have been obtained in a variety of clinical trials, and one virus (T-vec or Imlygic) has been FDA approved for the treatment of metastatic melanoma, and is thought to partially act through the induction of relevant immune responses.
Our lab was the first to develop a mathematical / computational framework to study the dynamics of oncolytic viruses in 2001, with the aim to investigate correlates of successful virus spread and to ultimately predict testable treatment regimes that improve outcome. Since then, this area of research has gained a large number of investigators, with many studies building on the mathematical foundations that we established.
Recently, we studied the very early dynamics of virus spread in a spatially structured population of cells in vitro, because such early dynamics remained poorly understood and are likely crucial for determining the long-term outcome of oncolytic virus infection. In collaboration with experimental virologist Dr Hung Fan at UC Irvine, we applied spatially structured computational models to data on virus spread using a GFP-labeled virus that replicated on a 2-dinensional monolayer of 293 cells with agar layover. Two distinct patterns of viral spread were observed under identical conditions. About 50% of growth foci in culture displayed a “robust growth pattern”, characterized by a plaque-like expansion of the infected cells. The other infection foci resulted in “limited growth”, characterized by a relatively slow infected cell expansion, followed by growth cessation. Using a mathematical-experimental approach, we found that these results can be explained by the occurrence of a race between virus spread and the spread of an interferon-induced antiviral state, where stochastic dynamics determine whether the infection moves into the domain of attraction of the robust growth outcome, or into the domain of attraction of the limited growth pattern. This work has defined important correlates of initial robust virus spread at the earliest stages of spatially explicit dynamics, which are likely key for successful outcomes of oncolytic virus therapy.


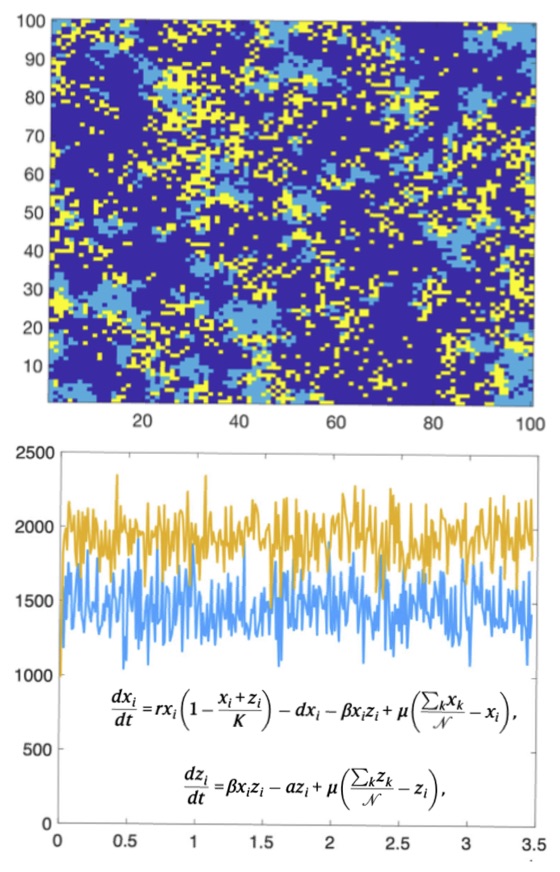
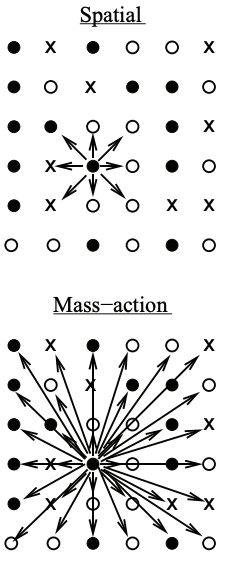
Evolutionary theory related to diseases
In addition to the modeling that is done in close collaboration with experimental and clinical laboratories, my lab further develops more general evolutionary theory related to diseases. One such example is our work describing the spatial dynamics of fitness valley (or fitness plateau) crossing. This refers to the evolution of an advantageous phenotype that requires the accumulation of two or more mutations, where each individual mutation confers a disadvantage, or is neutral, compared to the wild-type. This is relevant to a variety of disease-related scenarios, for example to the emergence of growing cancer cells, which can require multiple mutational hits, each of which alone can be neutral or disadvantageous compared with healthy cells in the tissue. The theoretical literature has examined the rate at which fitness valleys are crossed in a variety of different settings. Cancer cell populations, however, evolve in the context of pronounced spatial structures. We provided a comprehensive analysis of the spatial fitness valley / plateau crossing dynamics using a constant-population Moran process model and a non-constant population contact process model. We found that spatial interactions accelerate the crossing of fitness valleys in the Moran process in the context of neutral and disadvantageous intermediate mutants, because of the formation of mutant islands that increase the lifespan of mutant lineages. By contrast, in the contact process, spatial structure can accelerate or delay fitness valley crossing, and there can even be an optimal degree of mixing that maximizes the rate of evolution. These results demonstrate how evolutionary dynamics are affected by spatial interactions, which can modify the rate of evolution in a systematic way.
Another example is our work on mutant evolution in expanding, spatially structured populations. This is again highly relevant to solid tumors, where disease-driving and passenger mutants emerge in a growing, spatially structured cell population. Adding to previous work, we provided a simple derivation of scaling laws that characterize the number of mutants of different relative fitness in expanding populations in spatial models of different dimensionalities. These laws are universal and independent of “microscopic” modeling details. We also studied the accumulation of mutants. Similar to previous work, we found that with advantageous and neutral mutants, more of them are present in spatially structured, compared to well-mixed colonies of the same size. In contrast, we discovered that the behavior of disadvantageous mutants was different. Depending on whether the disadvantage was manifested through decreased division rates or increased death rates, the accumulation of mutants was shown to happen faster or slower in spatially expanding colonies, compared to their well-mixed counterparts. Last but not least, we showed that in all cases, the same results are observed in fragmented, non-spatial patch models. This suggests that the patterns observed are the consequence of population fragmentation, and not spatial restrictions per se.